Think about what you shared with your friends on Facebook today. Was it feelings of “stress” or “failure”, or perhaps “joy”, “love” or “excitement”? Each time we post on social media, we leave traces of our mood.
Our emotions are valuable commodities, and many companies are developing automated tools to recognise them in a process known as sentiment analysis.
Recently, a leaked report revealed that Facebook can identify when young people are feeling vulnerable, although the company has insisted it did not use the analysis to target users with advertising. Facebook also apologised in 2014 for an experiment on “emotional contagion” in which posts with either “positive” or “negative” sentiment were filtered from users’ feeds.
Clearly, the ability to detect emotion from text is of great interest to social media companies, as well as advertisers. But how does sentiment analysis work, why is it useful and what are the dangers?
How does sentiment analysis work?
While the details of Facebook’s own algorithm are not publicly known, most sentiment analysis techniques fall into two categories: supervised or unsupervised.
Supervised methods rely on labelled data. In other words, these are posts that have been classified manually as containing positive or negative sentiment.
Statistical methods are then used to train models to classify new posts automatically based on the presence of pre-identified words or phrases, for example “stressed” or “relaxed”.
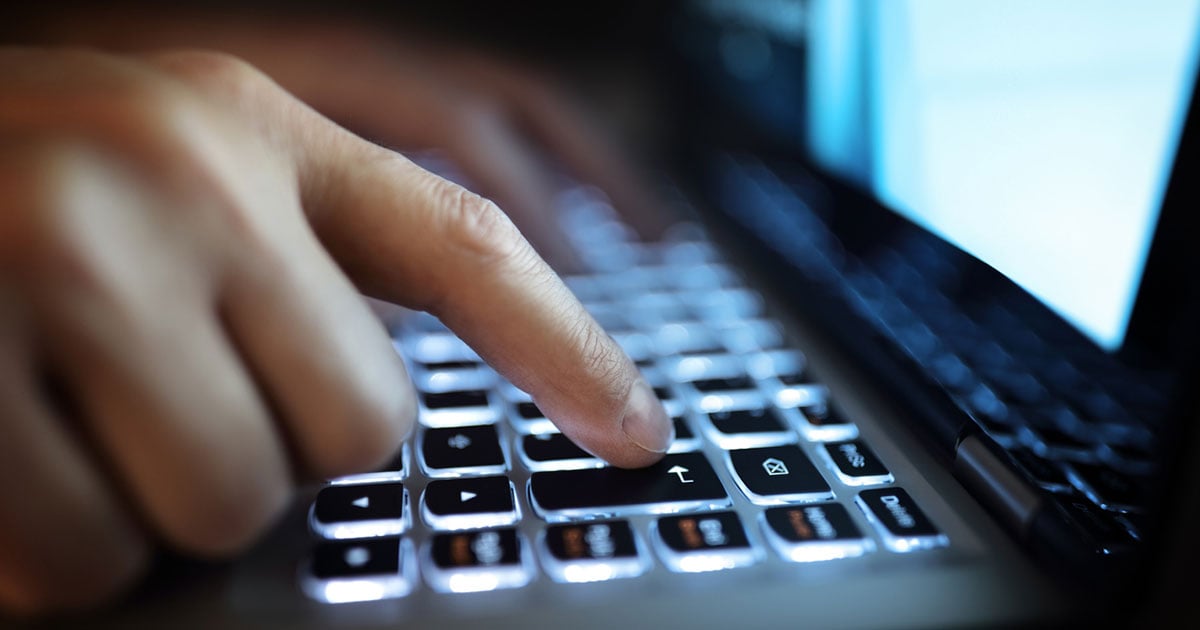